Spatio-temporal graph convolutional networks
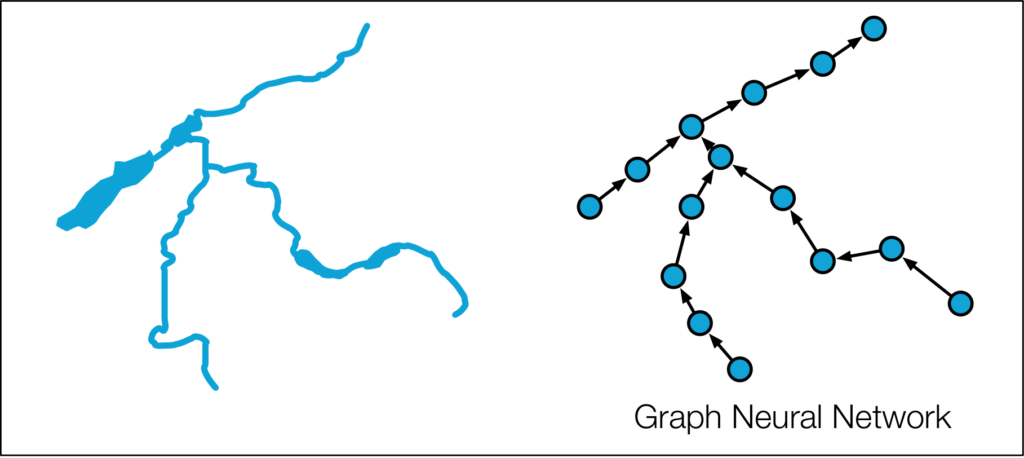
Time | 2022 — 2026 |
Funding | Swiss National Science Foundation (Practice-to-Science project in collaboration with the Bern University of Applied Sciences) |
Researchers | Benjamin Fankhauser, Kaspar Riesen |
Abstract: The Federal Office for the Environment (FOEN) analyses several environmental aspects of Switzerland. The monitoring of water temperatures over long time periods belongs to one of the most important tasks of the Hydrology Division of FOEN. At the moment, the Hydrology Division maintains approximately 80 metering stations. Stations measure various parameters such as water temperature, discharge, water level etc. The sampling frequency is in most cases consistently regulated at a ten minutes interval. Although the initiation date of monitoring varies between 1971 and 2015 as more stations were installed over time, concise water data is available over several decades. Besides the federal metering stations, the cantons of Switzerland maintain more than 700 additional stations. As is often the case in Switzerland, measurement policies vary in the different cantons.
Ongoing climatic change and thereby resulting adverse effects to all lifeforms and society are a major concern in almost all countries. Since several years rising river water temperatures are being observed. Hence, several studies have been conducted to model and forecast the river temperatures. As far as we could identify none of the current studies focus on the connectivity of rivers or try to model a large part if not the entire Swiss river (water) network as a collective. We propose a novel deep learning approach to modelling the Swiss river network with a spatio-temporal graph. Spatio- temporal graphs are graph structures where the node and/or edge features are allowed to change over time. Using the temporal information of the spatial graph, temperature for a future time step can be forecasted. The key idea of graph spatial-temporal networks is to consider spatial dependency and temporal dependency simultaneously. In this project we develop novel and robust methods, algorithms, data structures, and heuristics that go beyond current understanding in graph-based machine learning on a concrete physical system that is fundamentally important to our society.