Novel State-of-the-Art Graph Matching Algorithms
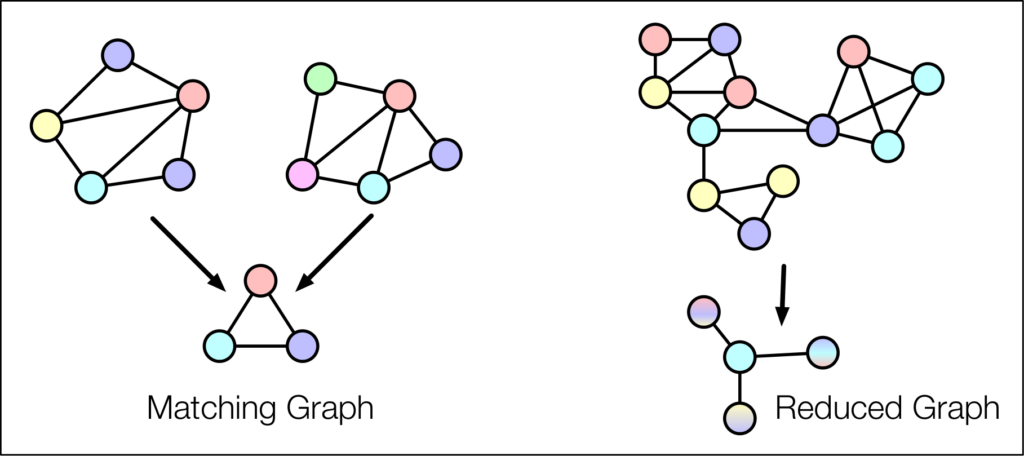
Time | 2020 — 2024 |
Funding | Swiss National Science Foundation |
Researchers | Anthony Gillioz, Mathias Fuchs, Kaspar Riesen |
Abstract: Due to fast developments in both storage media and data acquisition, we observe rapidly increasing amounts of data available in diverse areas in both science and industry. Simultaneously, we observe that in many applications the underlying data is inherently complex, making graphs the most useful and adequate data structure available to date. These two developments evoke the need for ongoing research of robust and efficient methods that assist humans in understanding and handling their pools of big sets of graph data.
A large amount of graph based methods for pattern recognition and related fields have been proposed. One of these methods is graph edit distance – a powerful and flexible graph dissimilarity measure and actually one of the main subjects of this proposal. Regarding graph edit distance (or more generally graph matching) we observe two substantial gaps in research that we aim to research and bridge. Formally, within the present project we will research (a) encodings of matching information in a novel data structure to formalize the stable cores of specific classes by means of graphs. The rationale of this matching-graph representation is that it can be beneficial to focus on stable/important parts of graphs during algorithmic comparisons (rather than on complete graphs). (b) hierarchical graph representations in conjunction with linear time graph embedding. This procedure is motivated by the fact that hierarchical representations (including fast and expressive graph embeddings) can be exploited in filter-and-verify strategies in order to substantially speed up and improve the matching processes.
By verifying both hypotheses we plan to make significant advances in the field of structural pattern recognition and establishing novel paradigms that go beyond the current understanding. In particular, the overall objective is the development and research of novel, robust graph edit distance methods that outperform the current state-of-the-art in graph matching on existing and novel data sets stemming from different real world scenarios. Hence, the proposed project involves both research on fundamental algorithms and solving concrete problems in applications. Last but not least, we aim at thorough comparisons of structural methods with recent deep learning methods in order to gain insights on where graph based methods actually rank to date.